yusukaid's IT note
Pytorch를 이용한 YOLO v3 논문 구현 #3 - weights file 이해하기 본문
※본 포스팅은 아래 블로그를 참조해 번역하고 공부한 것입니다.
https://blog.paperspace.com/how-to-implement-a-yolo-object-detector-in-pytorch/
Tutorial on implementing YOLO v3 from scratch in PyTorch
Tutorial on building YOLO v3 detector from scratch detailing how to create the network architecture from a configuration file, load the weights and designing input/output pipelines.
blog.paperspace.com
weights 는 딥러닝에서 순차적인 방식으로 저장된 가중치를 포함하는 이진 파일이다. 가중치를 불러올 때는 주의할 점이 많은데, 어느 레이어에 포함되어야 하는지에 대한 설명이 없고 정수로만 저장되어 있다. 따라서 어떻게 가중치가 저장되고 어느 레이어에 속하는지 이해해야 한다.
- 가중치는 batch norm layer 또는 convolutional layer에 속한다.
- 이런 레이어에 대한 가중치는 똑같은 순서대로 저장된다.
- batch norm layer가 나타나면 bias가 없으나, 나타나지 않으면 공식 file로 불러와야 한다.
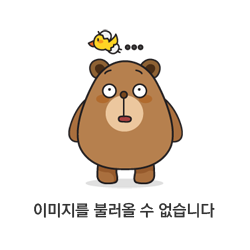
weights 불러오기
불러오는 가중치는 darknet 클래스의 멤버 함수가 된다.
def load_weights(self, weightfile):
#weights file 열기
fp = open(weightfile, "rb")
# 1. Major version number
# 2. Minor Version Number
# 3. Subversion number
# 4,5. 신경망에 의해 학습된 이미지 (training 도중)
header = np.fromfile(fp, dtype = np.int32, count = 5)
self.header = torch.from_numpy(header)
self.seen = self.header[3]
가중치 파일의 첫 160 byte는 파일의 헤더를 구성하는 5개의 int32 값들을 저장한다. 남은 bits들은 코드 순서대로 가중치를 표현하며, 이는 float32로 저장되어 있다.
weights = np.fromfile(fp, dtype = np.float32)
ptr = 0
for i in range(len(self.module_list)):
module_type = self.blocks[i + 1]["type"]
그후 남은 가중치는 np.ndarray로 불러오고, 해당 파일을 반복해 신경망의 모듈로 가중치를 불러온다.
#만약 module이 convolutional면 weights를 불러옴
#아닌 경우는 무시
if module_type == "convolutional":
model = self.module_list[i]
try:
batch_normalize = int(self.blocks[i+1]["batch_normalize"])
except:
batch_normalize = 0
conv = model[0]
convolutional이 batch_normalize를 포함하는지 확인하고, 그럴 경우 convolutional이라 판단해 가중치를 불러오는 로직이다.
if (batch_normalize):
bn = model[1]
#Batch Norm Layer의 가중치 수를 얻음
num_bn_biases = bn.bias.numel()
#weights 불러오기
bn_biases = torch.from_numpy(weights[ptr:ptr + num_bn_biases])
ptr += num_bn_biases
bn_weights = torch.from_numpy(weights[ptr: ptr + num_bn_biases])
ptr += num_bn_biases
bn_running_mean = torch.from_numpy(weights[ptr: ptr + num_bn_biases])
ptr += num_bn_biases
bn_running_var = torch.from_numpy(weights[ptr: ptr + num_bn_biases])
ptr += num_bn_biases
#불러온 weights를 모델 weights의 차원으로 변환
bn_biases = bn_biases.view_as(bn.bias.data)
bn_weights = bn_weights.view_as(bn.weight.data)
bn_running_mean = bn_running_mean.view_as(bn.running_mean)
bn_running_var = bn_running_var.view_as(bn.running_var)
#data를 model에 복사
bn.bias.data.copy_(bn_biases)
bn.weight.data.copy_(bn_weights)
bn.running_mean.copy_(bn_running_mean)
bn.running_var.copy_(bn_running_var)
만약 batch_norm이 true면 가중치를 불러온다.
#batch_norm이 false인 경우
else:
#biases 수
num_biases = conv.bias.numel()
#weights 불러오기
conv_biases = torch.from_numpy(weights[ptr: ptr + num_biases])
ptr = ptr + num_biases
#불러온 weights를 model weight의 차원에 맞게 reshape
conv_biases = conv_biases.view_as(conv.bias.data)
#data 복사
conv.bias.data.copy_(conv_biases)
반면 batch_norm이 false인 경우, 즉 bath norm-convolutional layer 그 어느쪽에도 속하지 않으면 convolutional layer의 biases를 불러온다.
#convolutional layer에 대한 weights 불러오기
num_weights = conv.weight.numel()
#weights를 위와 동일하게 함
conv_weights = torch.from_numpy(weights[ptr:ptr+num_weights])
ptr = ptr + num_weights
conv_weights = conv_weights.view_as(conv.weight.data)
conv.weight.data.copy_(conv_weights)
최종적으로 convolutional layer의 weights를 불러온다.
darknet.py는 이상으로 마무리입니다. 다음 챕터에서는 각종 유틸 함수를 최종 정리해보겠습니다.
'YOLO' 카테고리의 다른 글
Pytorch를 이용한 YOLO v3 논문 구현 #5 - input, output (0) | 2022.09.27 |
---|---|
Pytorch를 이용한 YOLO v3 논문 구현 #4 - NMS 구현과 objectness score (0) | 2022.09.27 |
Pytorch를 이용한 YOLO v3 논문 구현 #2 - 신경망 순전파 구현 (0) | 2022.09.27 |
Pytorch를 이용한 YOLO v3 논문 구현 #1 - 신경망 구조의 계층 생성 (22.10.04 수정) (0) | 2022.09.27 |
Pytorch를 이용한 YOLO v3 논문 구현 #0 - YOLO 구조 파악하기 (0) | 2022.09.27 |